Another month rolls by, and here at Jina AI we’re releasing a new, more refined algorithm for SceneXplain.
A while back, we introduced SceneXplain’s “Glide” algorithm, which nailed recognizing multilingual text in images, something other models are now only just introducing:
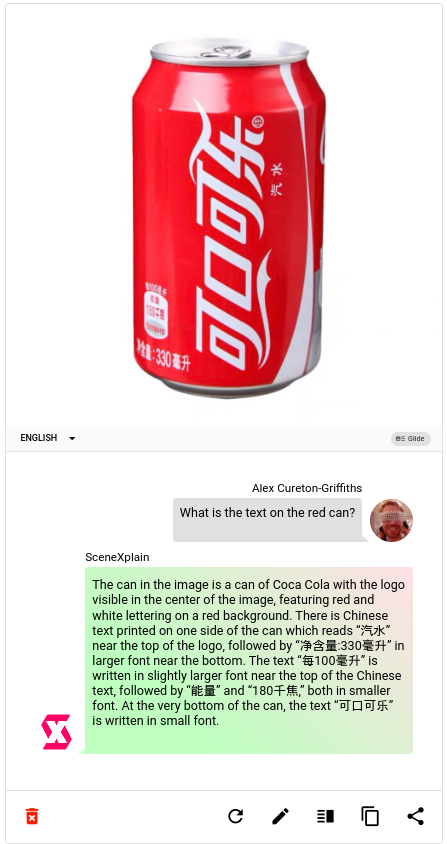
But that was then. This is now. So, what comes next? Fixing hallucination, that’s what.
tagWhat is hallucination in computer vision?
You may already be familiar with AI hallucination when it comes to LLMs, for example, ChatGPT hallucinating papers and sources that don’t exist in reality.
"[ChatGPT is an] omniscient, eager-to-please intern who sometimes lies to you"
Professor Ethan Mollick of Wharton
Likewise, image generation models (like Midjourney) often hallucinate unwanted details (or unwanted fingers for that matter.)
In short, hallucination is AI models generating false information.
SceneXplain often fell victim to this in our prior algorithms, hallucinating skateboarders, and occasionally Naruto:
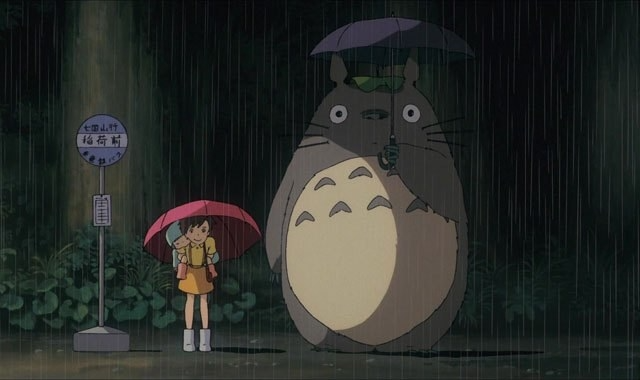
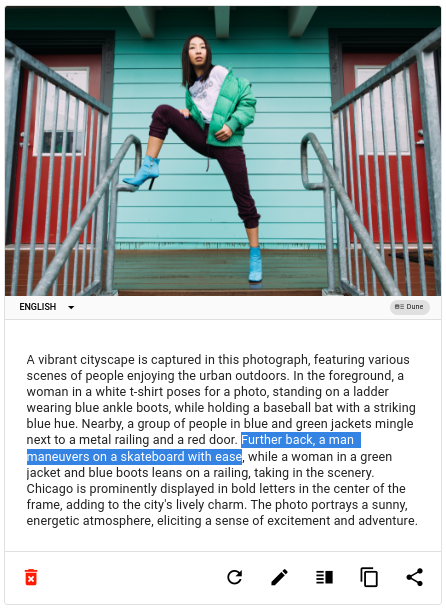
tagWhat causes hallucination in computer vision?
In humans, hallucination is caused by errors in perception, faulty expectations, neurological defects, or maybe just a dose of Scarecrow’s fear gas. In computer vision, it can often come about through issues with training data or the way an image is processed before generation or (in the case of SceneXplain) captioning.
tagSlicing and dicing: the elephant in the room
When it comes to image captioning, you often want a high level of detail. A common approach is to slice an image into several pieces, generate a caption for each piece, then merge the captions together into a final description. Sounds like a pretty sensible approach, right? By focusing on each segment and then combining results, we should get a better description overall.
Except...you ever heard the tale of the blind men and the elephant?
Six blind men encounter an elephant for the first time and each touches a different part of the animal. One feels the trunk and thinks it's a snake, another touches a leg and believes it's a tree, and so on with the tusk (a spear), ear (a mat), tail (a rope), and side (a wall). Amidst much debate, they all insist they're correct, not realizing they're all describing the same elephant.
If we want to understand an image by slicing it into different parts we can face exactly the same issue. If we were to draw what the blind men experience, we'd create a picture of a snake wrapped in a blanket next to a tree, along with a bunch of other elements. In short, many elements, but no elephant.
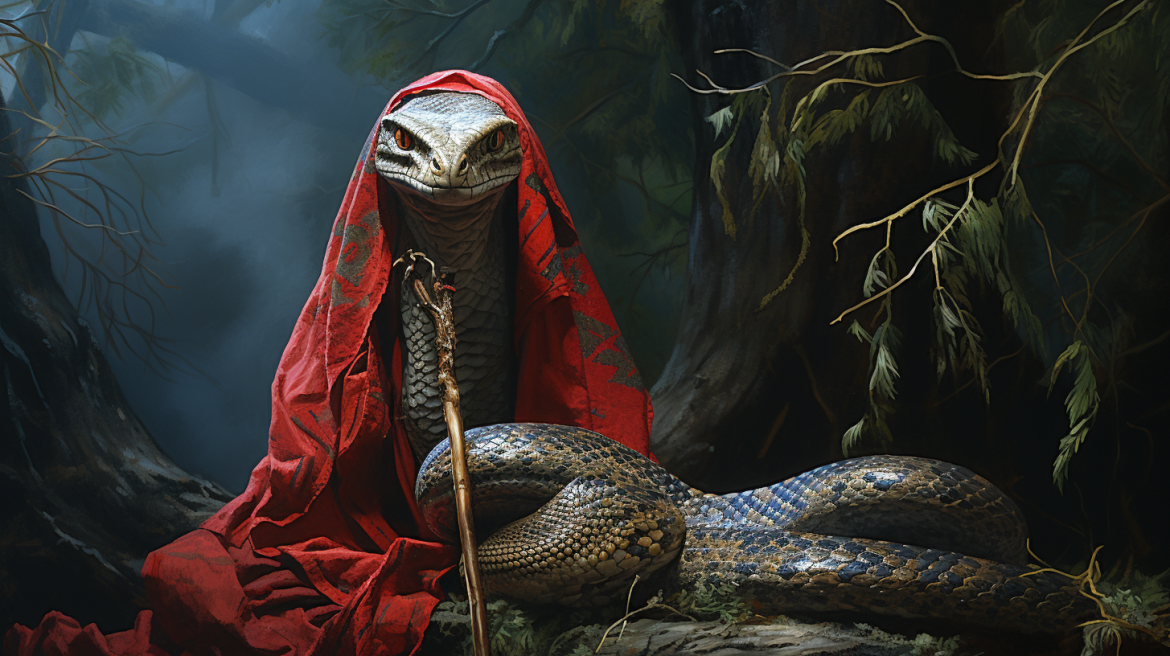
Likewise, in captioning, let's say we split up one image of three men into several segments. In each segment, there may be some part (arm, leg, side of head) of a different man. When it comes time to combine those segment descriptions (a man's face
, a man's leg
, a man's arm
, etc), how many men would be seen? Are these all part of one man? Are they each a part of different men? If so, how many? And without the context of the rest of the image, just what is that patch of fuzz in the top-left section?
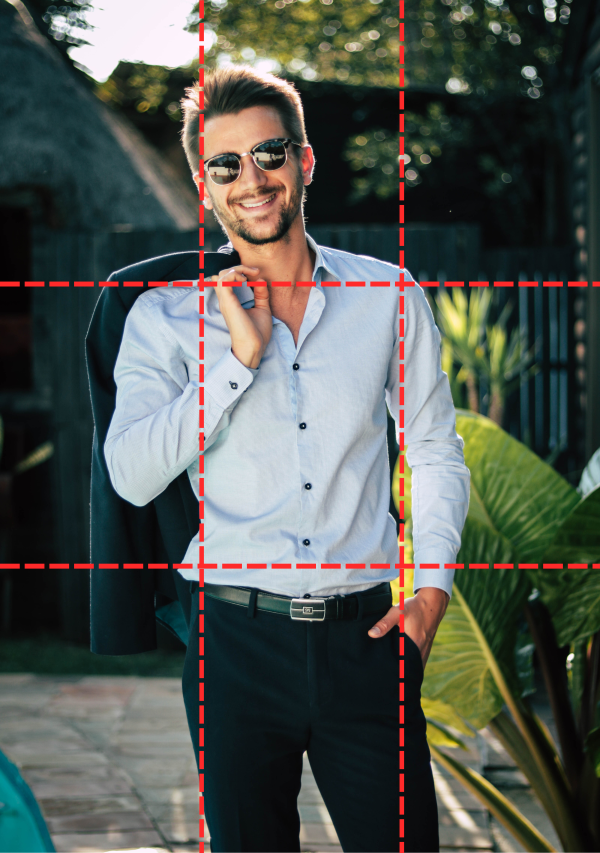
If the model splits everything up too much, it can hallucinate things that just aren't there. If it doesn't split things, the overall description may be too vague to be useful.
tagAmbiguity: That's a cute...puppy?
Many models rely on multimodality - that is translating text-to-image (like using a prompt to generate an image in Midjourney) or image-to-text (like generating a caption from an image in SceneXplain). Ambiguous text or images can therefore cause problems.
Take the phrase "salmon swimming in a river." Most humans would imagine fish swimming up a river. AI on the other hand can see some ambiguity:
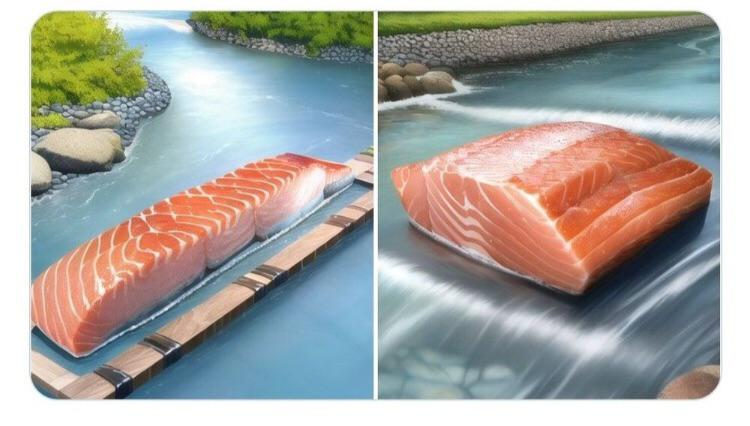
Likewise, sometimes images can be ambiguous:
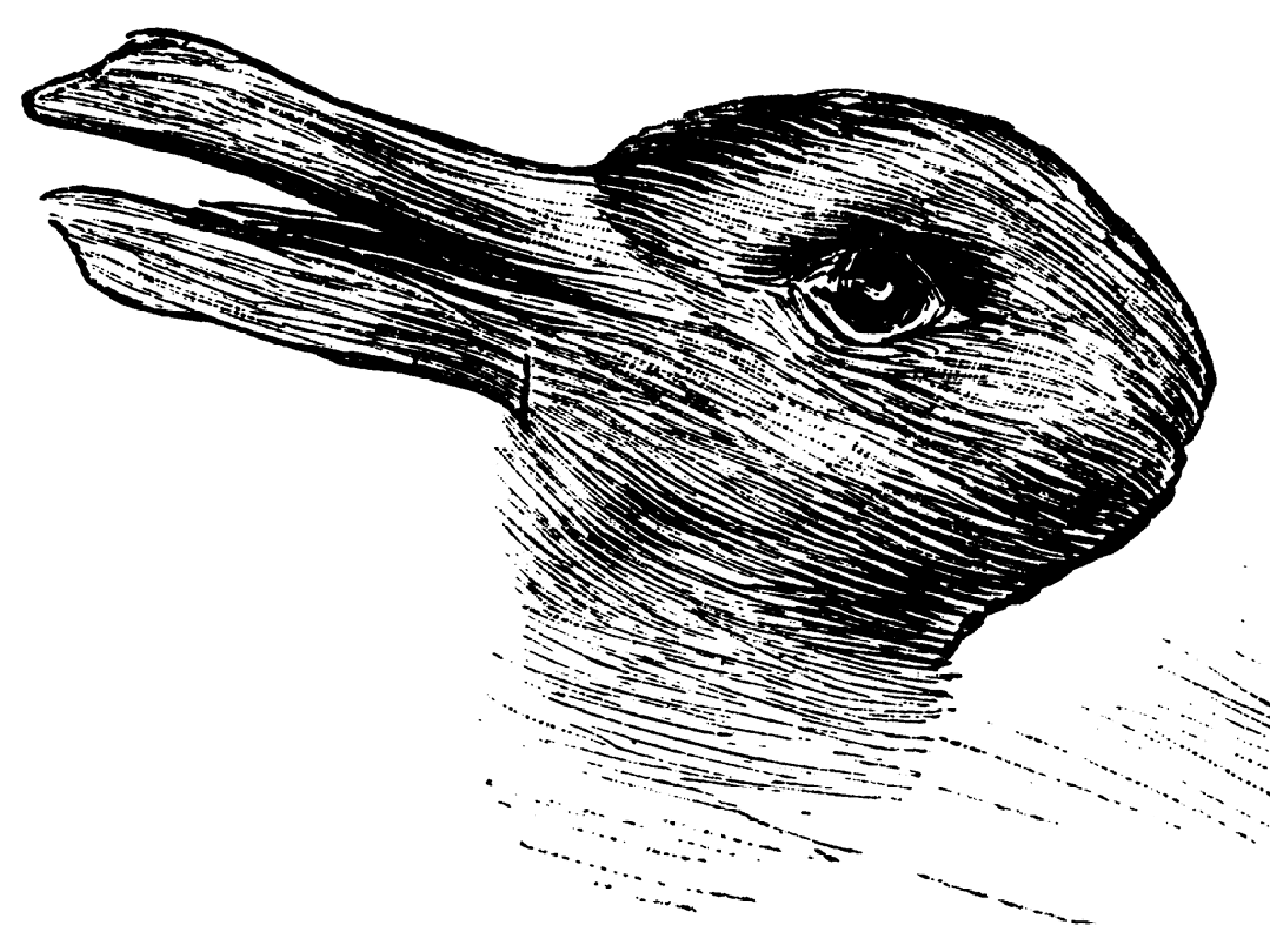
This isn't just applicable to traditional optical illusions or Rorschach tests. A cute little fuzzball could be a puppy OR a kitten. Running it by the model just once may result in a misclassification. Several tries may be needed to consistently see that puppy as a puppy.
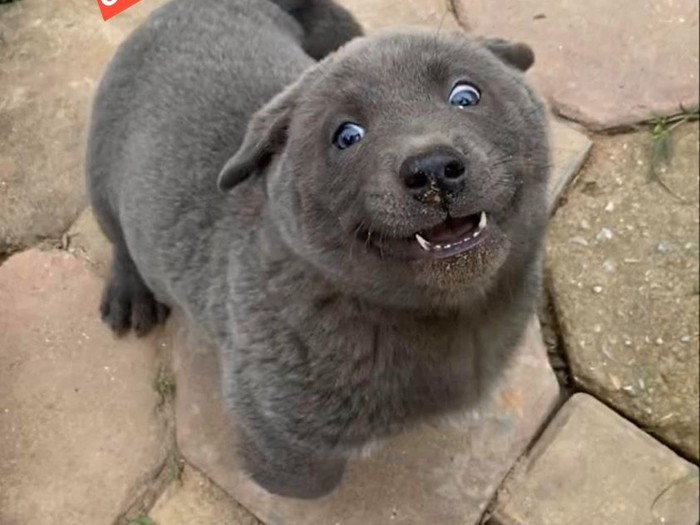
And let’s not even get started on that damn dress.
tagOCR: Occasionally Crappy Recognition
If you've got this far in the article, you probably think you're a pretty hot reader. Go on, give yourself a big pat on the back. You should really share some of your knowledge on expertsexchange.com. It's a site I use every day, sometimes several times. To exchange information with other experts.
It's not just a lack of clear word spacing that can trip you up. Take keming (uh, kerning) for example.
Bad kerning can have interesting results:
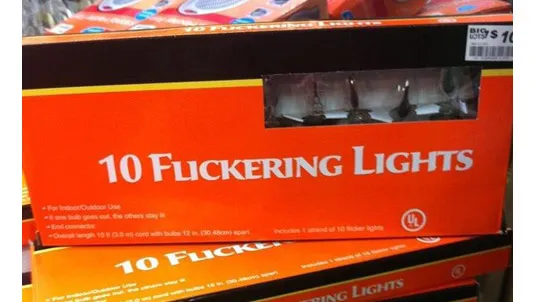
If these things are ambiguous to readers like you, just imagine how confused an AI model can get!
tagHow does SceneXplain fix hallucination?
SceneXplain’s new Jelly algorithm aims to eliminate these hallucinations, using the following methods:
- A simplified processing pipeline. Our prior algorithms had much more complex pipelines, meaning more places for things to go wrong. Jelly pares this back with a new end-to-end approach to image labeling that produces detailed image captions with much fewer hallucinations.
- Self-consistency: We’ve implemented an advanced prompt technique (self-consistency) to get the most consistent and accurate caption for any given image.
- OCR improvements: We've improved Jelly’s text recognition capabilities which leads to fewer hallucinations and better captions.
tagBefore and After
As you can see from the examples below:
- Glide suffers from way more hallucinations (highlighted in bold) than Jelly
- Jelly provides more readable captions, lacking the elaborate flowery language of Glide
tagLes Choristes
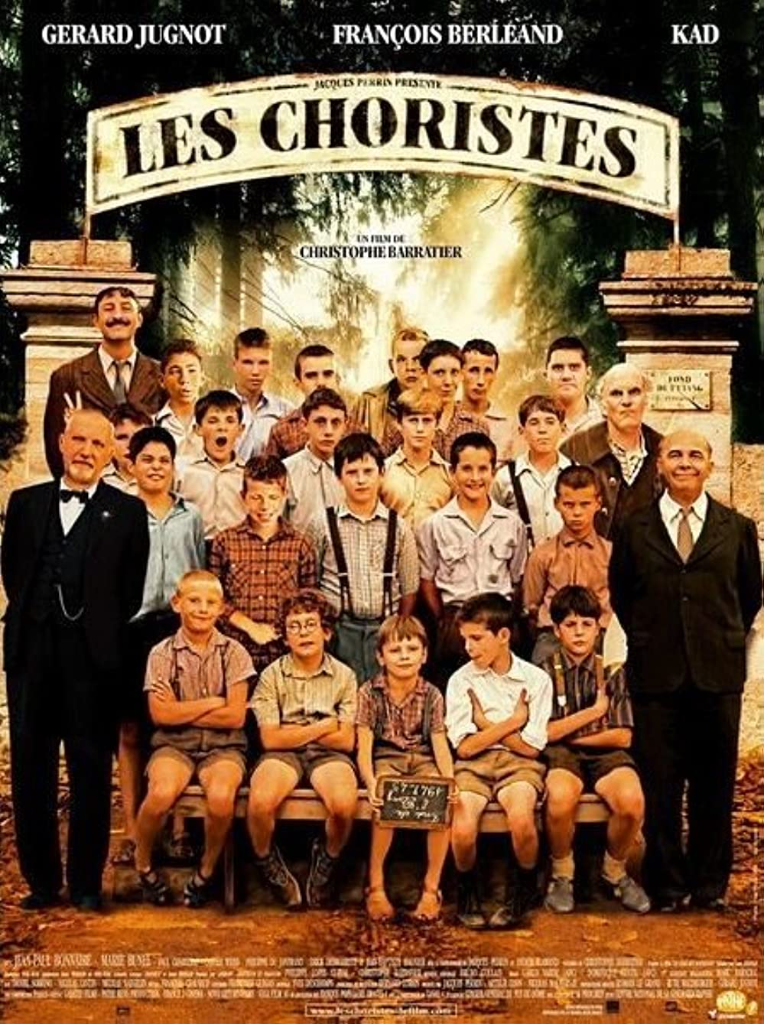
Glide:
The image showcases a poster for a movie called "Les Choristes." The poster features the title "LES CHORISTES" prominently displayed in the center of the image. The names of the actors and director, including "GERARD JUGNOT," "FRANÇOIS BERLEAND," "KAD," and "CHRISTOPHE BARRATIER," are listed below the title.
The poster shows a group of people posing for a picture in various settings. On the left side of the image, a group of people in tuxedos can be seen standing in front of a tree. In the middle of the image, a group of children sits on a bench with a chalkboard. To the right of the children, another group of children is sitting in a classroom. They are separated by a group of boys who are sitting on a bench. Further to the right, a group of people is posing for another picture. Above all these scenes, there is a group of children sitting on a stage.
The color scheme of the poster is predominantly white, with the title and texts in black. The actors' names are in smaller font compared to the title. The overall appearance suggests a heartwarming and collaborative movie involving children and adults in different settings, possibly related to music or education.
Additional details, such as the ratings "9.8" and "27.7.761," are included at the bottom right corner of the poster. The meaning of these numbers is unclear without further context.
Jelly:
The image is a movie poster for the French film "Les Choristes", directed by Christophe Barratier. The movie's title is printed in large letters under an archway. Beneath this are young boys and men, some sitting on a bench, and others standing. They are dressed in classic school-like clothing and are positioned at different heights, perhaps indicating different ages. In the lower corners of the poster, the names of actors Gérard Jugnot, François Berléand, and Kad are printed. The poster has a vintage feel, possibly due to its color scheme and the classic clothing of the characters.
tagLiberty leading the people
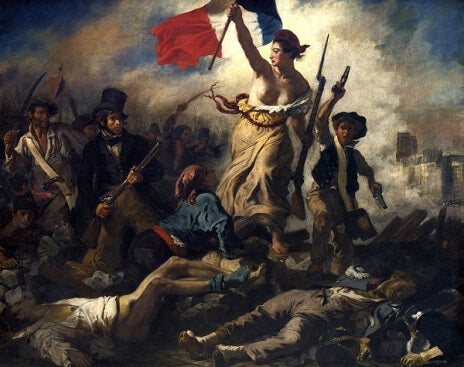
Glide:
The artwork depicts a scene with a strong patriotic theme. The central focus of the painting showcases a woman holding the French flag, her figure dominating the composition. Her proud stance and the vibrant colors of the flag capture the viewer's attention. Surrounding her, several other characters are depicted, each with their own distinct role. A man is shown holding a rifle, signifying the presence of armed conflict. In another section, a group of men is seen together on a boat, suggesting a collective effort or journey. Additionally, a man on a separate boat is featured, adding further depth to the narrative. A woman with a knife and a man with a gun are shown, reflecting a sense of danger and potential violence. The artwork overall conveys a sense of patriotism, conflict, and tension through its composition and subject matter.
Jelly:
This image is a painting titled "Liberty Leading the People", created in 1830 by the French artist Eugène Delacroix. The painting depicts a woman symbolizing France and the revolution, standing on the bodies of prostrate figures signifying defeated enemies. She is dressed in a blue dress, holding a French flag in her left hand, symbolizing the French republic and democracy. In her right hand, she is likely holding a weapon, possibly a sword. The painting represents a moment from the French Revolution, showcasing the spirit of the people resisting tyranny.
tagEmojis
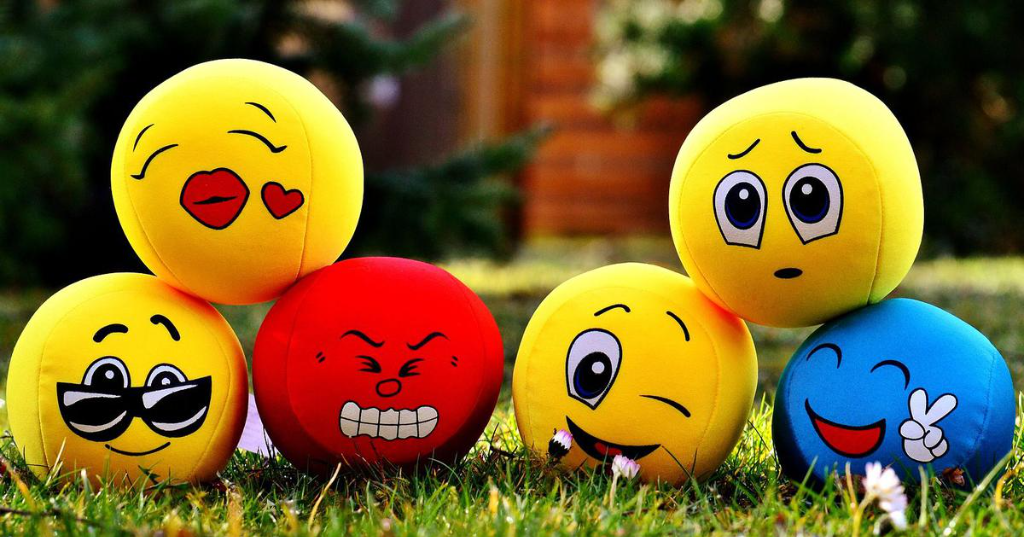
Glide:
A playful scene unfolds on a vibrant green grassy expanse, as a group of colorful emoji balls gathers together. These enchanting characters fill the frame, showcasing a wide array of expressive emotions and witty designs. In the center, a striking red and yellow ball stands out with its angry face emblazoned upon its surface. Positioned nearby, lying comfortably on the grass, two yellow emoji balls exude a relaxed demeanor. To the right, a buoyant blue and yellow ball features a cheerful smiley face that adds a touch of delight and cheerfulness to the mix. On the left, two yellow balls adorned with sunglasses mirror each other's fashionable style while resting peacefully in the grass. Amidst the lively atmosphere, a yellow and blue plush toy makes its presence known with a sad expression, attracting sympathy and affection. Additionally, a yellow and red emoji doll captivates attention with a heartfelt kiss imprinted upon its face. Further accentuating this whimsical scene, a lone yellow face with big expressive eyes captures the viewer's gaze, radiating a sense of curiosity and intrigue. Lastly, a yellow teddy bear wearing sunglasses and a friendly smile completes the array of delightful characters, inviting joy and warmth into the playful world they inhabit.
Jelly:
The image shows a group of six stress balls with different emoji faces on them, situated on a green grassy surface. Each stress ball displays a unique emoji expression and they come in varied colors including yellow, red, and blue. The background of the image is blurred, ensuring the focus is on these stress balls.
Want to see more examples of how SceneXplain algorithms stack up? Check them out here:
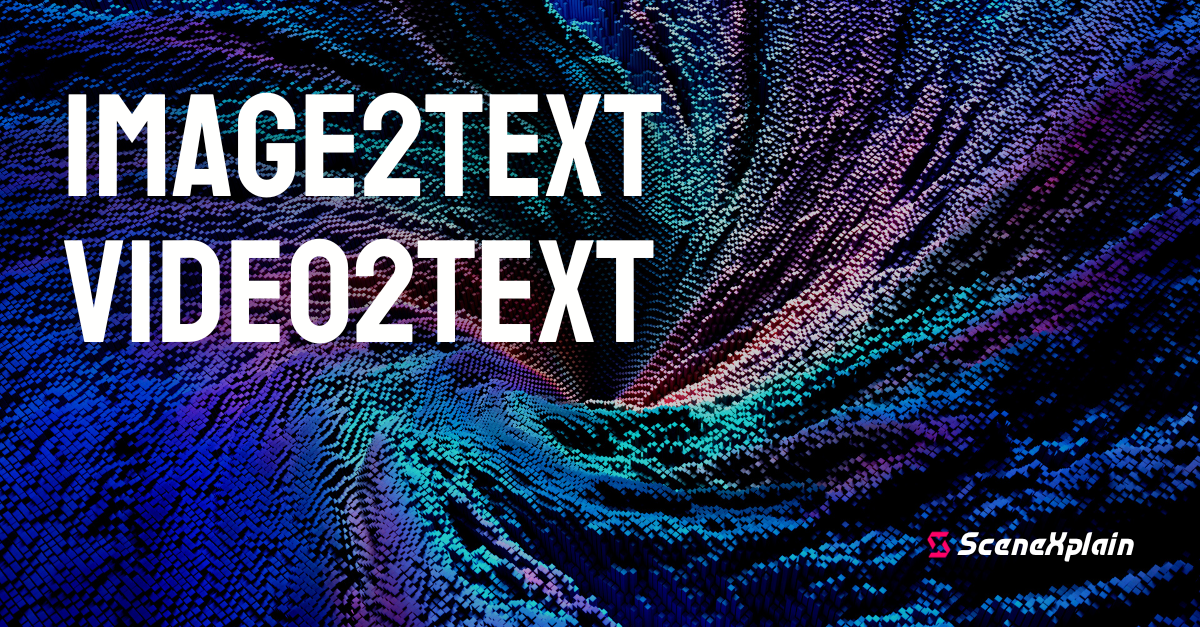
tagGet your Jelly on
As you can see, Jelly gives you captions that are more concise, readable, and accurate than ever before. Wave goodbye to hallucinations and say hello to detailed, precise descriptions of your images.
To get started with Jelly (or any other SceneXplain algorithm), sign up for a free account at scenex.jina.ai and start captioning your images!
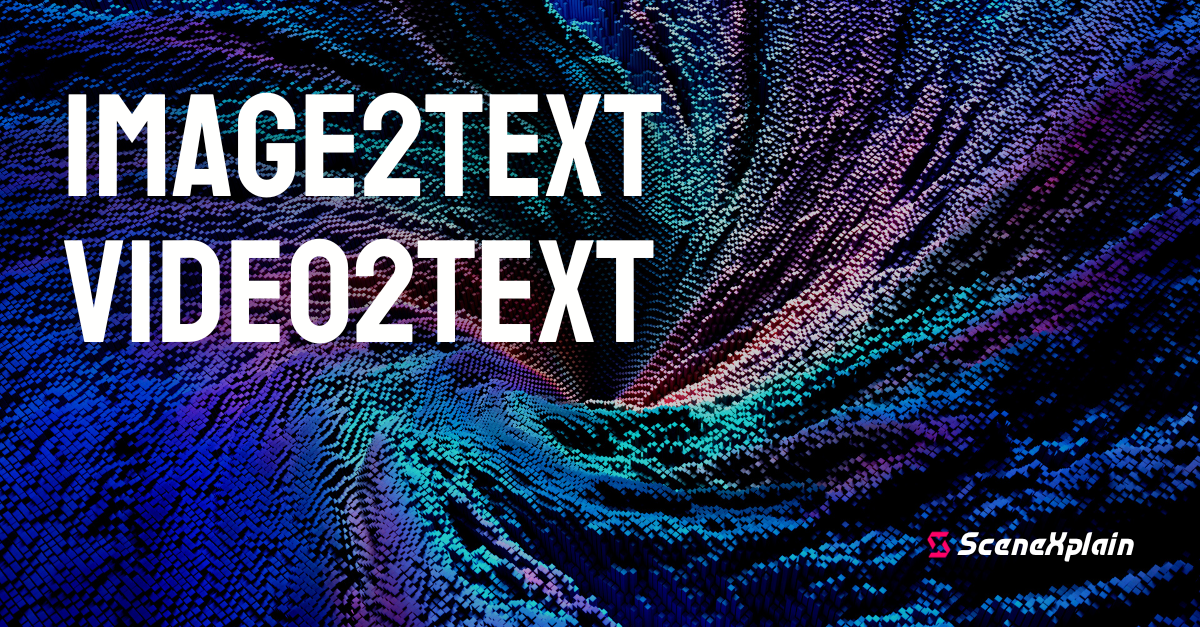