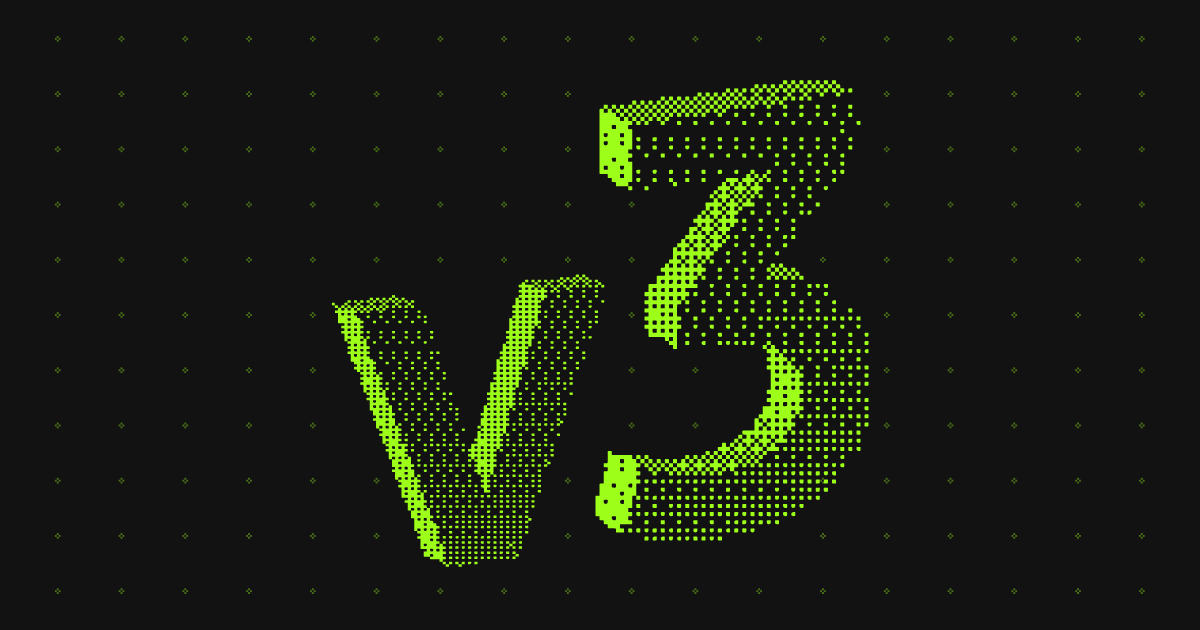
jina-embeddings-v3 has been released on Sept. 18, 2024. The best <1B multilingual embedding model.
Berlin, Germany - January 15, 2023 – Echoing JFK's iconic 'Ich bin ein Berliner', at Jina AI we're thrilled to bridge languages in our own way. Today, we're proud to announce our latest innovation: jina-embeddings-v2-base-de, a German/English embedding model. This state-of-the-art bilingual model is a significant stride forward in language representation, boasting a context length of 8,192 tokens. What sets it apart is its remarkable efficiency: it achieves top-tier performance while being only 1/7th the size of comparable models.
Embeddings are crucial for German businesses looking to expand into the U.S. market. According to the German American Business Outlook (GABO) 2022, approximately a third of German companies generate over 20% of their global sales and profits in the U.S., with 93% expecting an increase in U.S. sales. This trend continues as 93% plan to grow their company's U.S. investments in the next three years, with 85% expecting net sales growth and a significant focus on digital transformation. Good embeddings can play a pivotal role in this expansion by facilitating better understanding of customer preferences, enabling more effective communication, and positioning culturally resonant products.
Our breakthrough is particularly beneficial for German businesses looking to implement bilingual applications in English-speaking countries. With jina-embeddings-v2-base-de, we're excited to see how German companies will innovate and thrive in an increasingly connected world.
tagModel Highlights
- State-of-the-art Performance: jina-embeddings-v2-base-de consistently ranking at the top in relevant benchmarks and leading among open-source models of similar size.
- Bilingual Model: This model encodes texts in both German and English, allowing the use of either language as the query or target document in retrieval applications. Texts with equivalent meanings in both languages are mapped to the same embedding space, forming the basis for multilingual applications.
- Extended Context: An 8192-token length enables jina-embeddings-v2-base-de to support longer texts and document fragments, far surpassing models that only support a few hundred tokens at a time.
- Compact Size: jina-embeddings-v2-base-de is built for high performance on standard computer hardware. With only 161 million parameters, the entire model is 322MB and fits in the memory of commodity computers. The embeddings themselves are 768 dimensions, a relatively small vector size compared to many models, saving space and run-time for applications.
- Bias Minimization: Recent research shows that multilingual models without specific language training show strong biases towards English grammatical structures in embeddings. Embedding models should be about capturing meaning and not favor sentence pairs that are merely superficially similar.
- Seamless Integration: Jina Embeddings v2 models have native integrations with major vector databases, including MongoDB, Qdrant, and Weaviate, as well as RAG and LLM frameworks such as Haystack and LlamaIndex.
tagLeading Performance in German NLP
We've put jina-embeddings-v2-base-de to the test against four renowned baselines that also support both German and English. These include:
- Multilingual-E5-large and Multilingual-E5-base from Microsoft
- T-Systems’ Cross English & German RoBERTa for Sentence Embeddings
- Sentence-BERT (
distiluse-base-multilingual-cased-v2
)
Our benchmarks include the MTEB tasks for English and our own custom benchmark. Given the lack of a comprehensive benchmark suite for German embeddings, we took the initiative to develop our own, inspired by the MTEB. We're proud to share our findings and breakthroughs with you here.
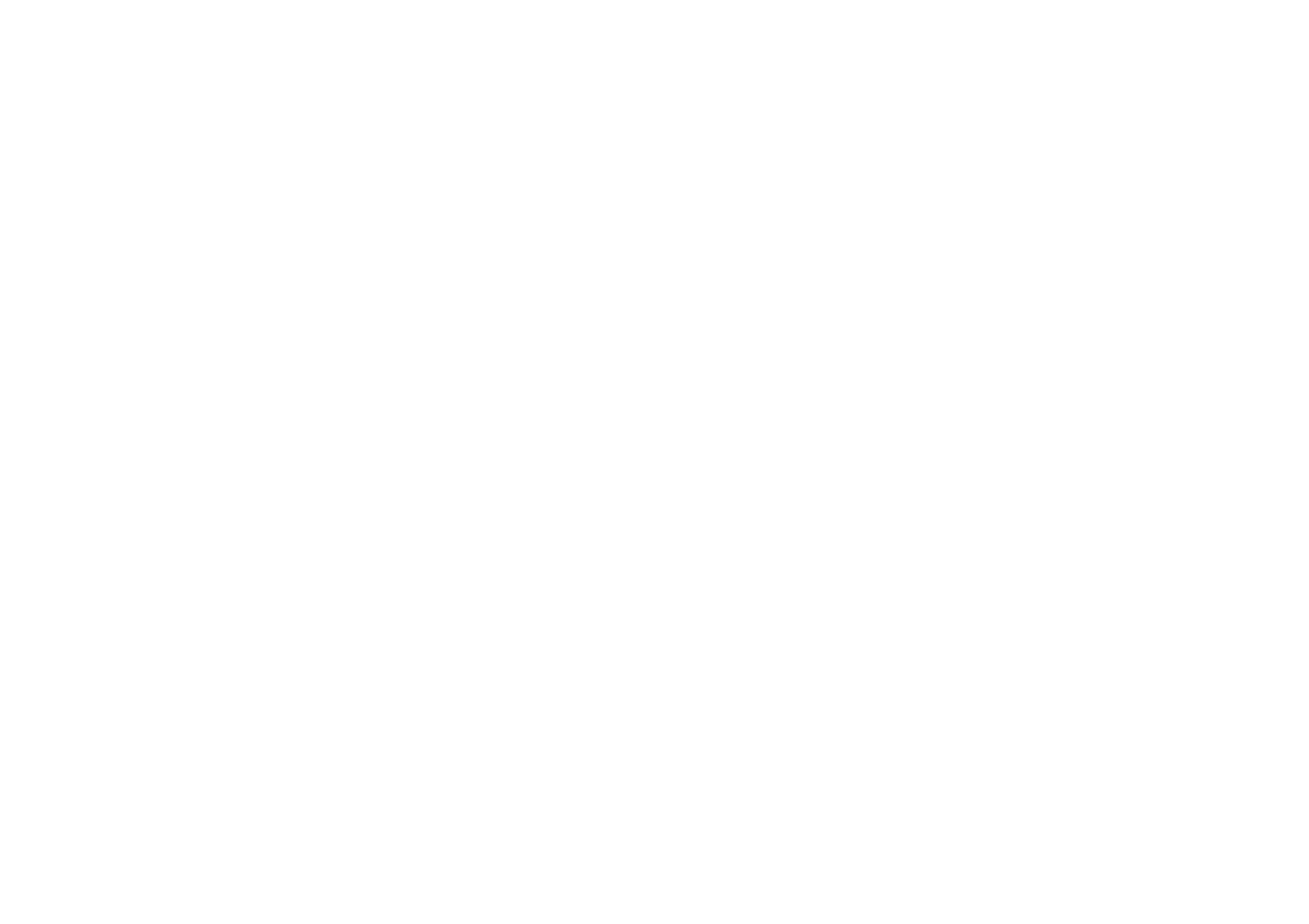
tagCompact Size, Superior Results
jina-embeddings-v2-base-de demonstrates exceptional performance, especially in German language tasks. It outshines the E5 base model while being less than a third of its size. Moreover, it stands toe-to-toe with the E5 large model, which is seven times larger, showcasing its efficiency and power. This efficiency makes jina-embeddings-v2-base-de a game-changer, particularly when compared to other popular bi- and multilingual embedding models.
tagExcelling in German-English Cross-Language Retrieval
Our model isn't just about size and efficiency; it's also a top performer in English-German cross-language retrieval tasks. This is evident in its performance in various key benchmarks:
- WikiCLIR, for English to German retrieval
- STS17, part of the MTEB evaluation for English to German retrieval
- STS22, for German to English retrieval, also part of MTEB
- BUCC, for German to English retrieval, included in MTEB
The performance in these benchmarks, particularly in the MTEB evaluation tests (with the exception of WikiCLIR), underscores the effectiveness of jina-embeddings-v2-base-de in handling complex bilingual tasks.
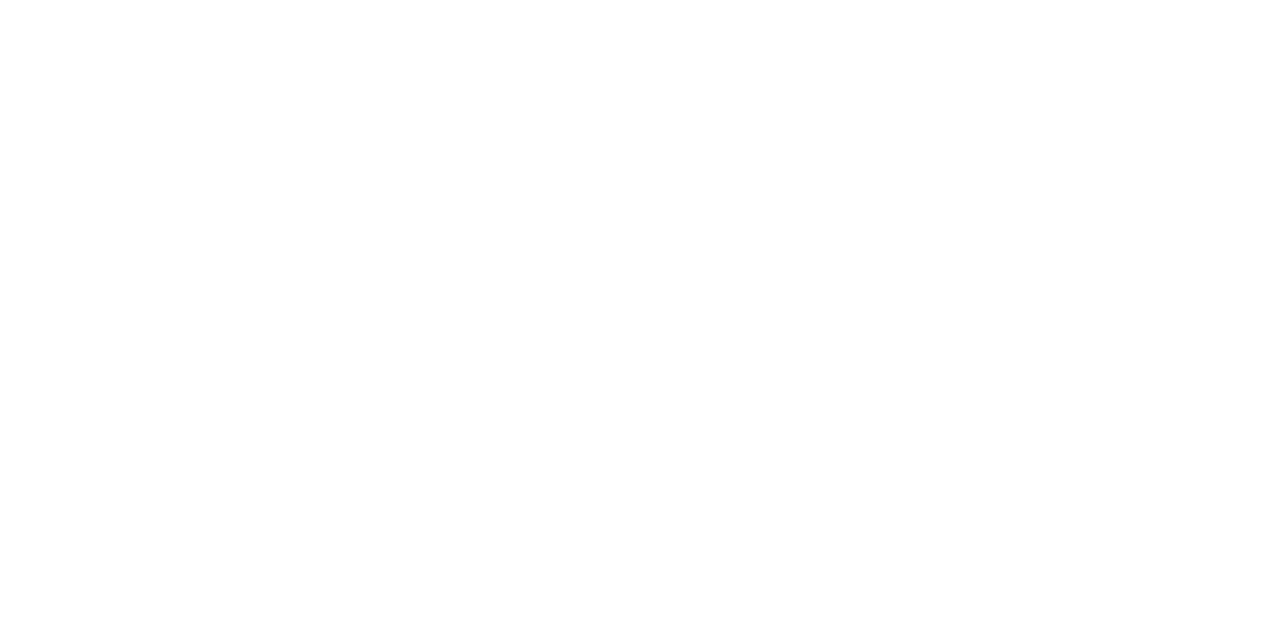
tagGet API Access
Our offerings for our enterprise users who value privacy and data compliance, including jina-embeddings-v2-base-de, are accessible via the Jina Embeddings API:
- Visit Jina Embeddings API and click on the model dropdown
- Select jina-embeddings-v2-base-de
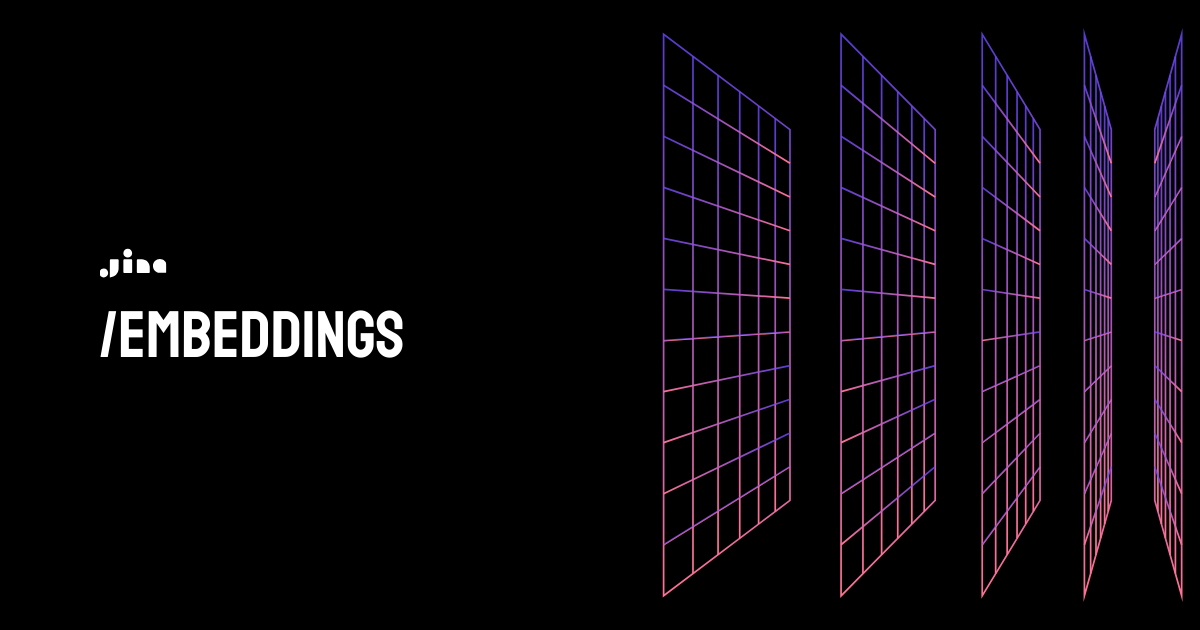
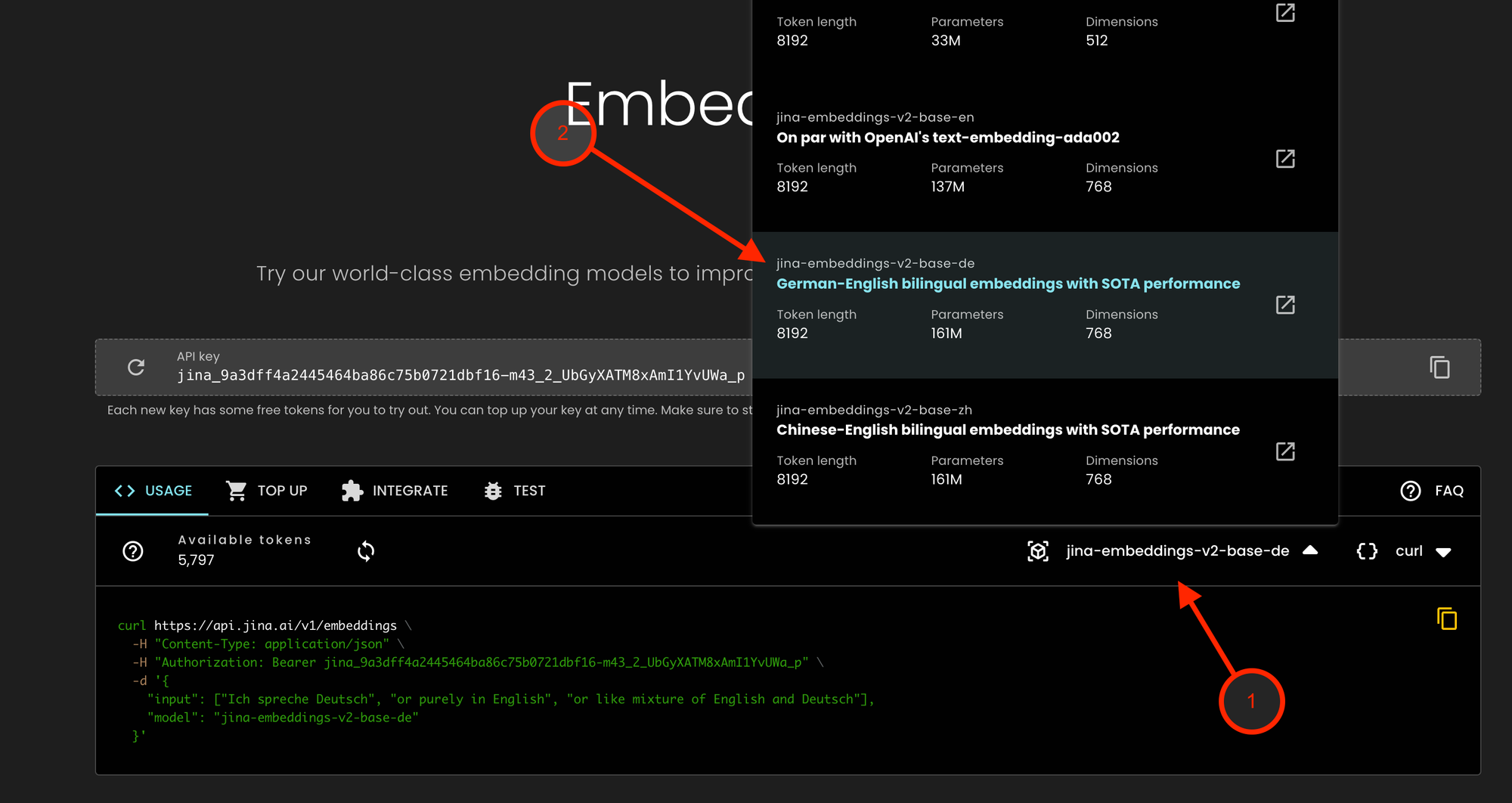
We will make this model available in the AWS Sagemaker marketplace for Amazon cloud users and for download on HuggingFace very soon.
tagJina 8K Embeddings: The Cornerstone of Diverse AI Applications
Embeddings are crucial for a wide range of AI applications, including information retrieval, data quality control, classification, and recommendation. They are fundamental to enhancing numerous AI tasks.
Jina AI is committed to advancing the state-of-the-art in embedding technology, keeping our core AI components transparent, accessible, and affordable to enterprises of all types and sizes that value privacy and data compliance. In addition to jina-embeddings-v2-base-de, Jina AI has released state-of-the-art embedding models for Chinese and high-performance English monolingual models. This is part of our mission to make AI technology more inclusive and globally applicable.
We value your feedback. Join our community channel to contribute feedback and stay informed about our advancements. Together, we're shaping a more robust and inclusive AI future.
