The advent of artificial intelligence has ushered in a new era of multimodal understanding, where image captioning algorithms play a critical role in interpreting and explaining complex visual information. These algorithms have evolved beyond generating simple captions, delving deeper into scene analysis to provide comprehensive descriptions of images. With the growing importance of these technologies in our increasingly connected world, the need for accurate and in-depth image understanding has never been more crucial.
The widespread use of image captioning technologies in various domains, such as digital accessibility, multimedia content creation, and data analysis, highlights their growing impact on our daily lives. As we venture into this comprehensive assessment, we aim to objectively analyze each algorithm's strengths and weaknesses, enabling you to make informed decisions based on their performance.
In this blog post, we thoroughly compare the leading image captioning algorithms in the market: SceneX, MiniGPT4, MidJourney /describe
, BLIP2, and CLIP interrogator 2.1. Our evaluation focuses on their ability to generate detailed, topical, factual, and readable descriptions for non-trivial images, emphasizing the significance of advanced scene understanding and explanation.
tagIntroducing the Contenders
This section briefly introduces each of the top image captioning algorithms included in our comparison. These algorithms showcase diverse techniques and approaches to achieve advanced scene understanding and explanation.
- SceneXplain: A state-of-the-art image captioning solution, SceneXplain harnesses the power of large language models like GPT-4 to generate sophisticated, detailed, and contextually rich textual descriptions for complex visual content. It aims to set the benchmark in the field by providing unparalleled image understanding.
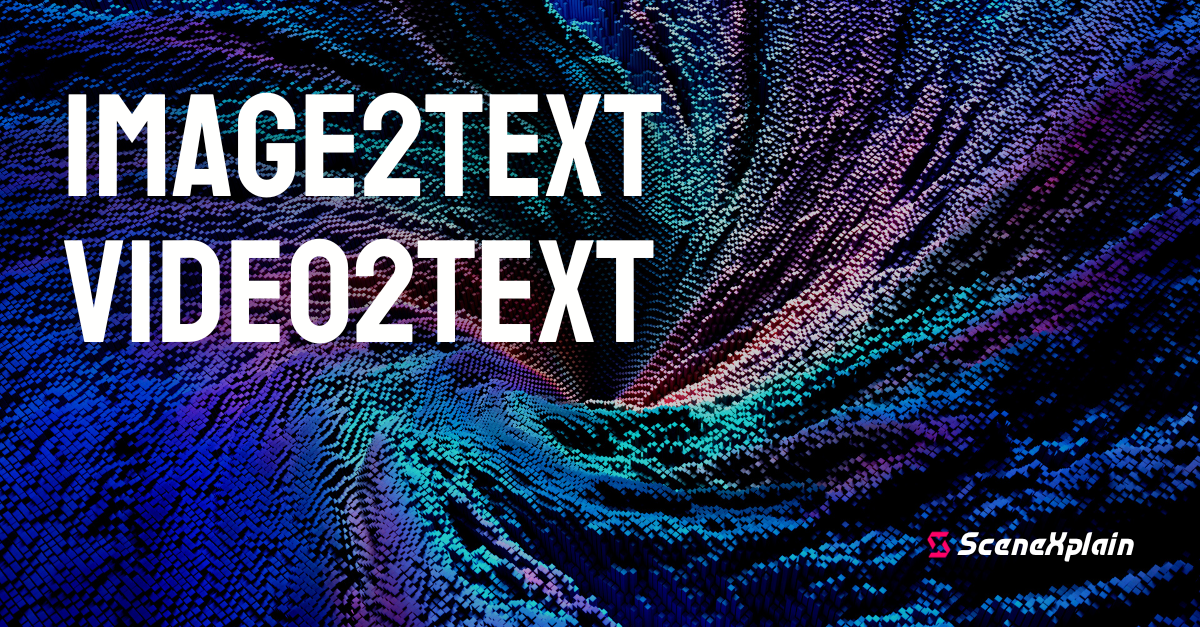
- MiniGPT4: A derivative of the remarkable GPT-4, MiniGPT4 aligns a frozen visual encoder with a frozen large language model (LLM), Vicuna, using a single projection layer. The model boasts many of GPT-4's advanced multi-modal generation capabilities, including detailed image description generation and website creation from hand-written drafts. By curating a high-quality dataset and using a conversational template for fine-tuning, MiniGPT4 addresses issues of unnatural language outputs and enhances the overall usability of the model.
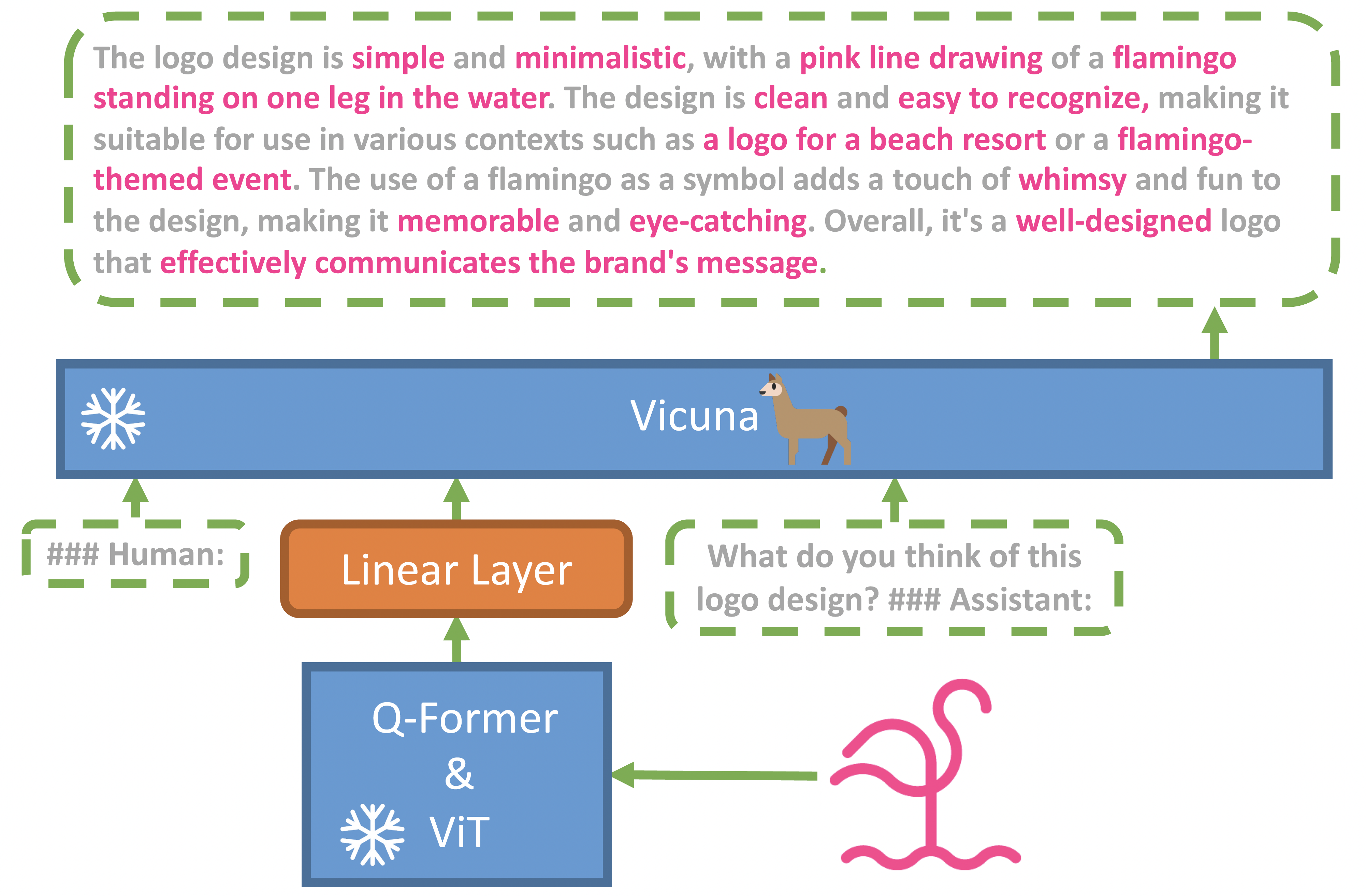
- Midjourney
/describe
: Recently introduced by Midjourney, the /describe command aims to transform images into prompts, augmenting the linguistic-visual process for both creative power and discovery. This feature is available inside the Midjourney Discord bot by calling/describe
.
BLIP2: A groundbreaking pre-training strategy, BLIP2 bootstraps vision-language pre-training using off-the-shelf frozen pre-trained image encoders and frozen large language models. With significantly fewer trainable parameters, BLIP2 achieves state-of-the-art performance on various vision-language tasks, highlighting its efficiency and effectiveness.
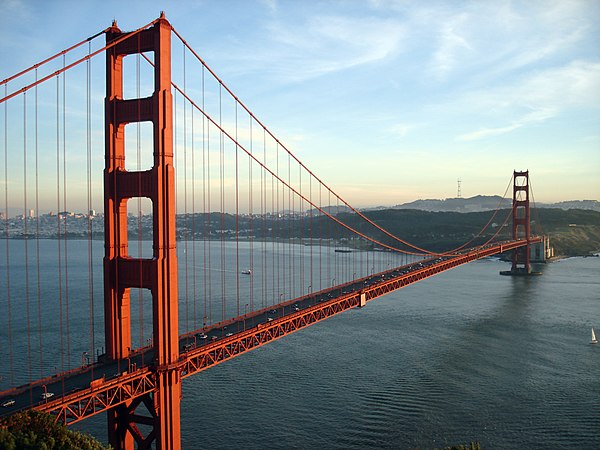
CLIP Interrogator 2.1: A specialized tool optimized for use with Stable Diffusion 2.0, the CLIP Interrogator 2.1 is designed to generate prompts for creating new images based on existing ones. Utilizing the ViT-H-14 OpenCLIP model, this algorithm demonstrates the potential for innovation in the image captioning domain.
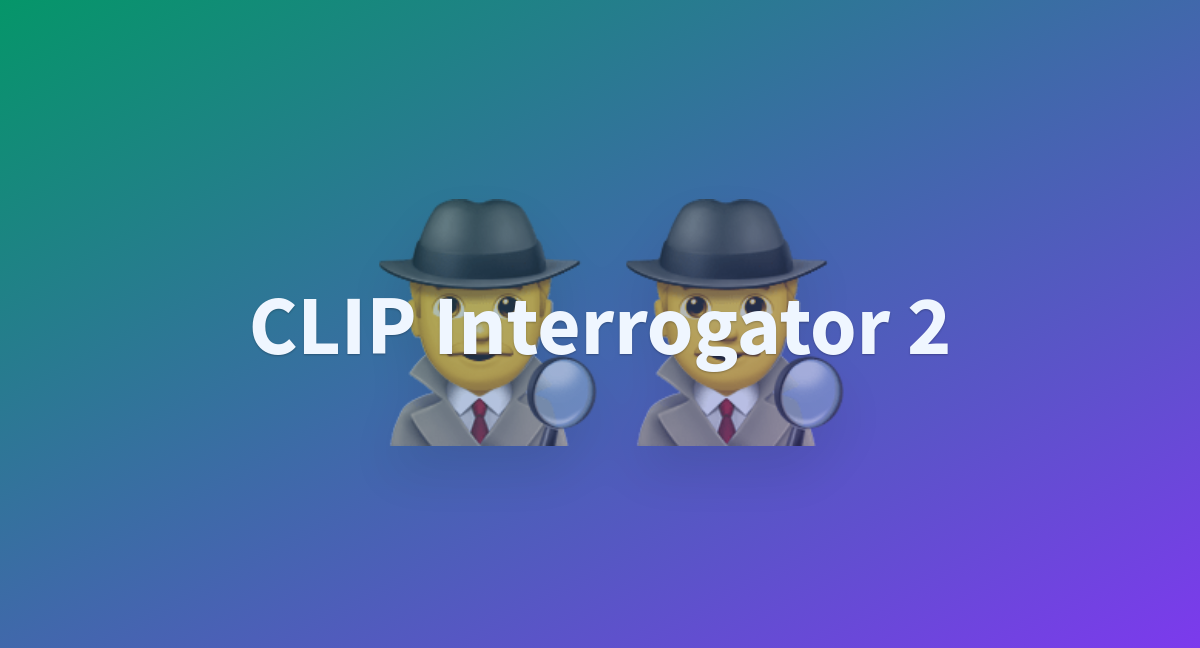
tagEvaluation Metrics
To ensure a fair and comprehensive comparison of the image captioning algorithms, we have selected a set of evaluation metrics that focus on the quality of generated captions.
tagDetails
This metric evaluates an algorithm's ability to capture and accurately describe every detail present in a complex scene. By assessing the level of granularity in the generated captions, we can determine how well an algorithm comprehends and conveys intricate visual information.
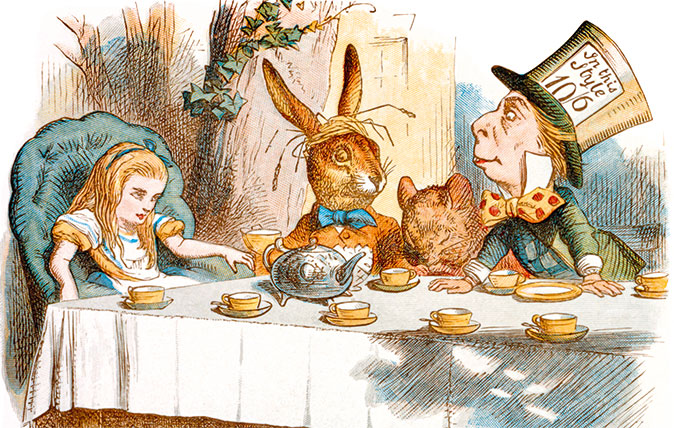
Given by SceneXplain/Comet (Score with 5): In this whimsical scene, a group of people and animals gather around a table for a delightful tea party. A young girl sits among her peculiar companions, which include a dapper man wearing a hat and bow tie, a cat with an inquisitive expression, and an adorable rabbit adorned with a blue bow tie. The table is laden with tea cups and teapots, adding to the charming atmosphere. This enchanting illustration transports viewers into an imaginative world where humans and anthropomorphic animals come together to enjoy each other's company over steaming cups of tea.
Given by BLIP2 (Score with 1): an illustration of alice and the rabbit at a tea party
tagTopicality
This metric measures an algorithm's ability to recognize and incorporate content related to contemporary events, popular culture, and well-known figures or works in its generated descriptions. Topicality reflects an algorithm's capacity to generate relevant and engaging captions that resonate with the intended audience.
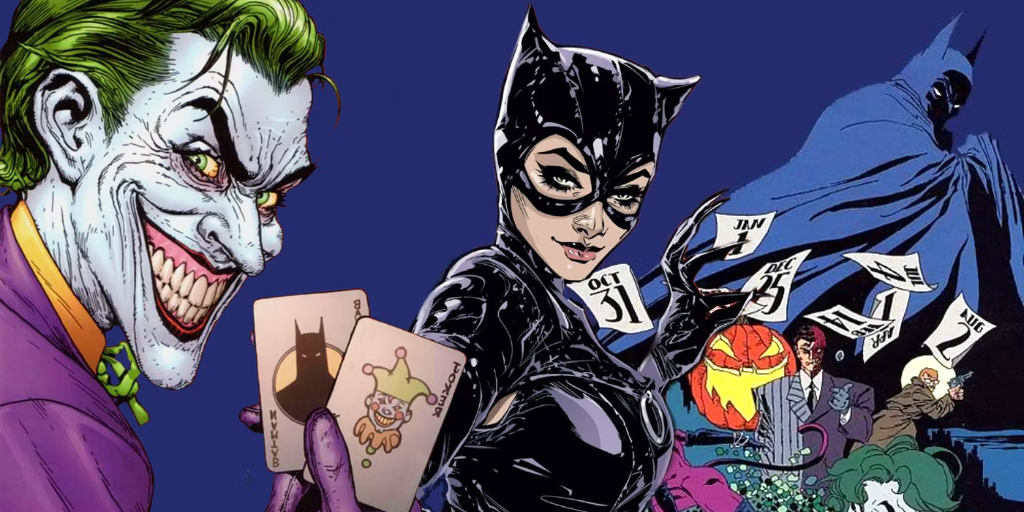
Given by SceneXplain/Bolt (Score with 5): In this captivating scene, the Joker and Batman command attention as they engage in a thrilling face-off. The Joker, with his signature grin and a card in hand, faces off against a woman donning a Batman costume. The tension between them is palpable, yet the atmosphere takes an unexpected twist as Batman and Catwoman are found playing cards together. The Bat-Symbol adorns Batman's head, signifying his unwavering dedication to justice. Amidst this intriguing tableau, numerous other characters from DC Comics populate the scene, infusing it with a sense of excitement and intrigue that keeps viewers on the edge of their seats.
Given by CLIP Interrogator (Score with 1): a group of comic characters standing next to each other, inspired by Jim Lee, serial art, cats playing poker, kylie jenner as catwoman, header, neal adams | centered
tagFactuality
Factuality evaluates an algorithm's adherence to reality while generating meaningful and factually correct content. The focus of this metric is to minimize hallucination or the generation of false information in the captions. High factuality scores indicate that an algorithm is able to consistently generate accurate and trustworthy descriptions of the images.

Given by SceneXplain/Dune (Score with 5): The devastating aftermath of Hurricane Katrina in the Bahamas is captured in this heartrending image. The once-thriving cityscape is now a scene of destruction, with numerous buildings reduced to rubble. An aerial view reveals a damaged bridge and a road marred by the powerful storm. Amidst the wreckage, houses lay in ruins, their once-sturdy structures now mere memories of a time before the hurricane's wrath. In the midst of this desolation, nature encroaches upon the remnants of civilization, with trees and foliage overtaking a house that stands alone in what was once a bustling community. The scene serves as a poignant reminder of the immense power and lasting impact of such natural disasters.
Given by MiniGPT4 (Score with 1): This image shows a view of a coastal town with houses and buildings on the shore. The town is surrounded by water and there are boats in the water. The houses are made of wood and have a thatched roof. The town is surrounded by trees and there are no people in sight. The sky is clear and there are no clouds. The image is taken from a high altitude and shows the entire town.
tagPerplexity
Correlated to readability, perplexity is a measure of how easy the generated text is to understand. Lower perplexity scores indicate that the text is more coherent and comprehensible, ensuring that users can quickly grasp the meaning behind the captions. This metric allows us to gauge the overall user experience provided by each algorithm in terms of readability.

It is important to note that we have chosen not to include speed as a metric in our evaluation, as it can be heavily influenced by factors such as GPU and infrastructure, which are outside the scope of our comparison. Our focus remains on the quality of the generated captions and their ability to provide advanced scene understanding and explanation.
To ensure an objective comparison of the performance of each image captioning algorithm across our chosen evaluation metrics, we have implemented a standardized scoring system, employing the expertise of external human labelers. These labelers, who are well-versed in the field of image captioning and scene understanding, were invited to independently assess the algorithms' performance. For each of the four metrics - Details, Topicality, Factuality, and Perplexity - the labelers assigned a score on a scale of 1 to 5, with 1 being the lowest and 5 being the highest. This approach guarantees that the scores are not only consistent across the various metrics but also free from any potential biases, ensuring a fair and reliable evaluation of the competing algorithms.
tagIn-Depth Analysis of Experimental Results
In order to evaluate the image captioning algorithms on a diverse and challenging set of images, we carefully selected a collection of 33 non-trivial, complicated images that encompass a wide range of styles, such as real-world scenes, artificial creations, oil paintings, photographs, and cartoons. This diverse dataset ensures that the algorithms are thoroughly tested on their ability to generate comprehensive descriptions for various types of visual content.
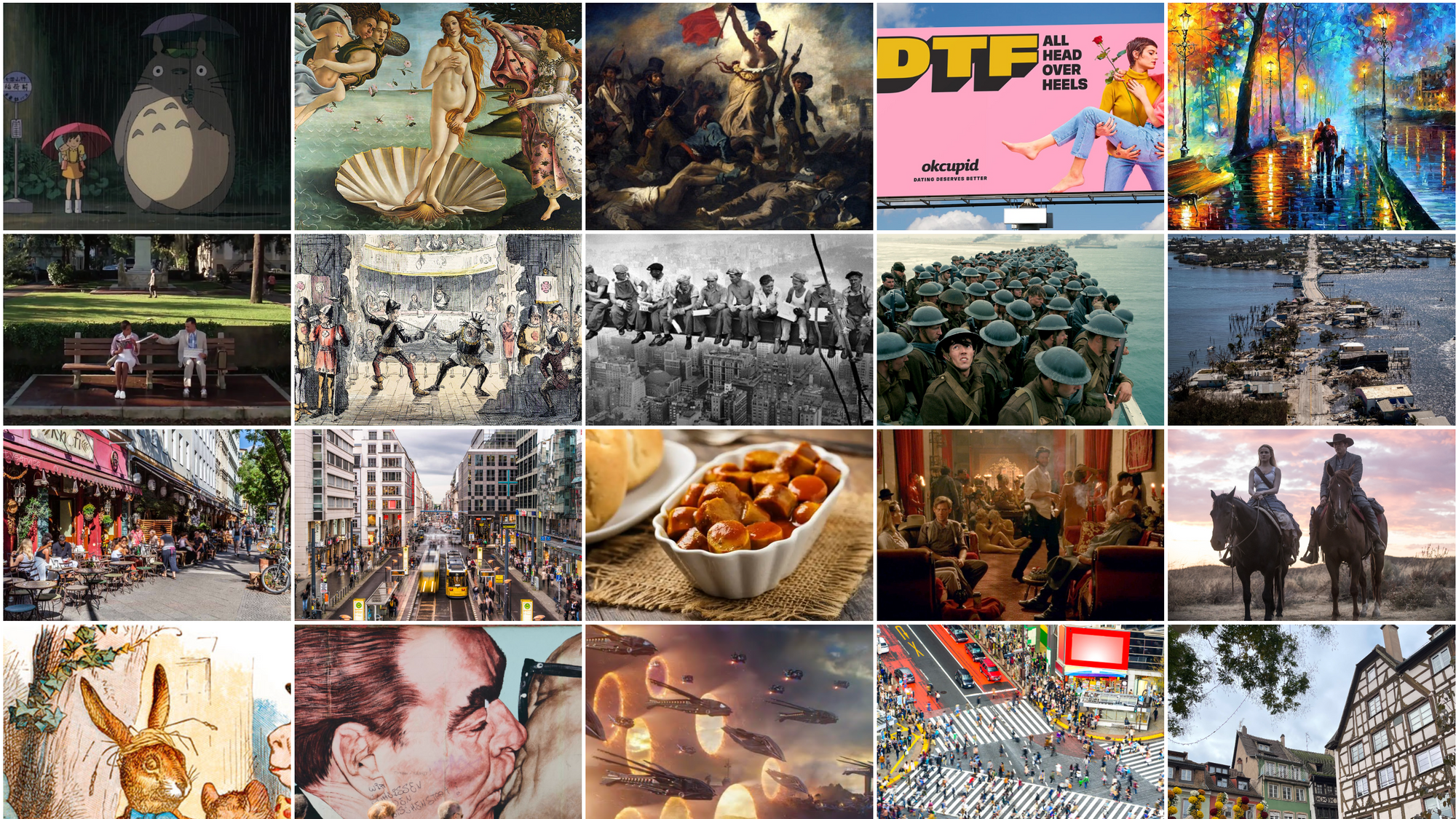
We have compiled the full results of our evaluation, including the captions generated by each algorithm, in a publicly accessible Google Spreadsheet. This detailed document allows interested readers to delve deeper into the performance of each algorithm and observe their image captioning abilities firsthand.
After evaluating each image captioning algorithm using the metrics discussed earlier, we have compiled the results in the following table:
Details | Topicality | Factuality | Perplexity | |
---|---|---|---|---|
SceneX - Aqua | 2.82 | 3.06 | 3.59 | 1.0281 |
SceneX - Bolt | 3.18 | 3.09 | 3.53 | 1.0275 |
SceneX - Comet | 3.30 | 1.70 | 3.38 | 1.0286 |
SceneX - Dune | 3.42 | 2.94 | 3.63 | 1.0288 |
Midjourney /describe |
1.61 | 2.21 | 3.03 | 1.1189 |
CLIP Interrogator 2.1 | 1.27 | 2.15 | 2.56 | 1.1554 |
BLIP2 | 1.00 | 1.76 | 4.00 | 1.7065 |
MiniGPT-4 | 2.97 | 1.59 | 3.33 | 1.0368 |
(Except for perplexity, other metrics are the higher, the better)
To provide a more intuitive visualization of the different algorithm characteristics, we have created a radar graph that displays the performance of each algorithm across the various metrics. This graphical representation allows for a clear comparison of their strengths and weaknesses, making it easier for readers to understand the differences in performance. However, we have decided to exclude the Perplexity metric from the radar graph, as its inclusion may not contribute to a clear interpretation of the area size. By focusing on the other metrics, the area size in the radar graph serves as a more effective indicator of each algorithm's overall performance in terms of detail, topicality, and factuality.
The experimental results of the image captioning algorithms provide valuable insights into their performance across various metrics. To better understand the underlying factors contributing to these results, we delve deeper into the challenges of image captioning and scene understanding and the potential reasons behind the differences in performance.
tagComplex visual information processing
SceneX variants (Aqua, Bolt, Comet, and Dune) consistently outperform the other algorithms in the Details metric, indicating a superior ability to capture and describe intricate visual information in the images. This suggests that SceneX is well-equipped to handle complex scene understanding and explanation.
The ability to accurately capture intricate details in an image is a challenging task. It requires the model to possess a deep understanding of the visual content and the capability to generate coherent and contextually appropriate textual descriptions. SceneX's consistent performance in the Details metric can be attributed to its advanced architecture that leverages the power of large language models, enabling it to handle complex scene understanding and explanation more effectively than its counterparts.
tagTopical relevance and engagement
SceneX - Bolt and SceneX - Aqua demonstrate the highest topicality scores among all algorithms, reflecting their capacity to generate relevant and engaging captions that resonate with contemporary events, popular culture, and well-known figures or works.
Generating captions that resonate with contemporary events, popular culture, and well-known figures or works is another difficult aspect of image captioning. This requires the model to have a broad knowledge base and the ability to contextualize and integrate such information in the captions. SceneX's superior performance in Topicality can be explained by its advanced training process, which likely exposes the model to a diverse range of topics and allows it to generate more engaging captions.
tagFactuality and hallucination
Although BLIP2 achieves the highest Factuality score, it falls short in other metrics, particularly Details and Topicality.
Ensuring factuality in the generated captions is crucial, as it helps avoid generating false information or hallucinations. One possible reason for BLIP2's high Factuality score but lower performance in other metrics could be that its focus on generating very concise sentences. On the other hand, SceneX strikes a balance between factuality and other metrics, which contributes to its overall strong performance.
tagReadability and perplexity
SceneX - Dune and SceneX - Aqua exhibit the lowest (best) perplexity scores, indicating that their generated captions are more readable and coherent than those of the other algorithms.
Achieving low perplexity scores, indicating high readability and coherence, is a challenge that requires the model to generate captions that are both contextually appropriate and easy to understand. SceneX's success in this metric can be attributed to its use of large language models, which are known for their ability to produce more coherent and natural-sounding text.
tagSceneXplain vs. miniGPT4
MiniGPT-4 performs relatively well in the Details and Factuality metrics, but it lags behind SceneX in terms of Topicality and Perplexity. This suggests that while MiniGPT-4 is a strong contender, it may not provide the same level of advanced scene understanding and engaging captions as SceneX.
While the results demonstrate the overall strength of the SceneX image captioning algorithm, it is crucial to maintain a subtle, objective perspective in our analysis. By examining the performance of each algorithm across all metrics, we can provide a comprehensive and unbiased assessment that allows users to make informed decisions based on their specific needs.
tagConclusion
Our comprehensive evaluation of various image captioning algorithms reveals that SceneXplain consistently delivers outstanding performance in terms of detail, topicality, factuality, and readability. By leveraging the power of advanced large language models like GPT-4, SceneXplain is able to generate sophisticated, contextually rich textual descriptions for complex visual content, setting it apart from its competitors.
The implications of such advanced image captioning capabilities are far-reaching, with several key areas in which SceneXplain can have a significant impact:
- Enhancing accessibility: Comprehensive image descriptions generated by SceneXplain can significantly improve the online experience for visually impaired users who rely on screen readers and image alt tags to navigate the digital world.
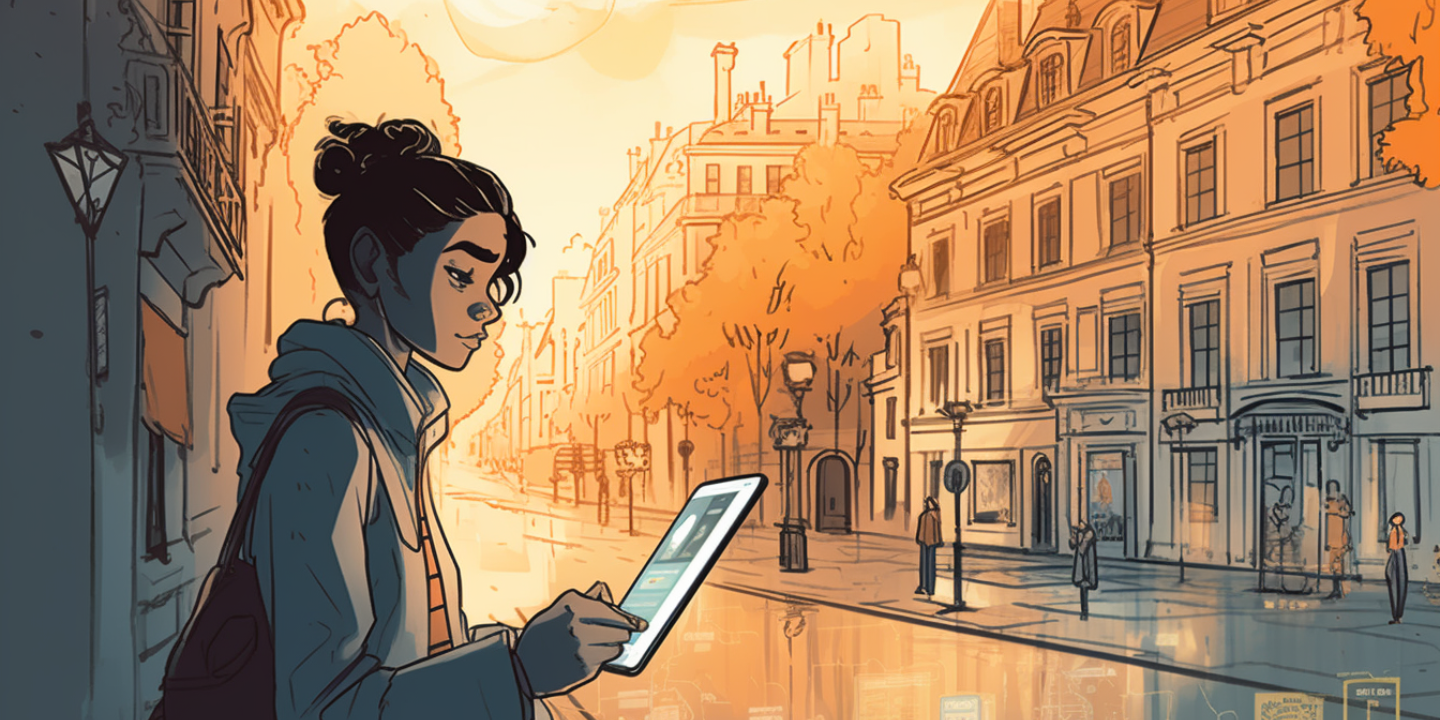
- Boosting SEO: Detailed and accurate image captions provided by SceneXplain can help search engines better understand visual content, potentially leading to improved rankings and increased organic traffic to websites.
- Elevating visual storytelling: The engaging and vivid descriptions generated by SceneXplain can add depth and richness to visual content, transforming ordinary images into immersive storytelling experiences.
As digital accessibility and multimedia content continue to gain importance, adopting cutting-edge solutions like SceneXplain is crucial for staying ahead in the rapidly evolving online landscape. While the performance of SceneXplain in this evaluation is noteworthy, we encourage readers to explore its capabilities and potential applications to appreciate its value fully.